EVENT
Event News
Project colloquium by Asst. Prof. Taro Sekiyama: "Towards automated proof synthesis for intuitionistic propositional logic with deep learning"
Date:
June 13 (Wed.)
Time:
16:30-18:00
Place:
ERATO MMSD Takebashi Site Common Room 3
Abstract:
Taro Sekiyama (National Institute of Informatics),
Towards automated proof synthesis for intuitionistic propositional logic with deep learning I report ongoing work on proof synthesis for intuitionistic propositional logic using deep learning. My talk consists of two parts.
The first is an application of an off-the-shelf deep neural network (DNN) model for machine translation tasks to proof synthesis. The idea of this work is to view the proof synthesis problem as a translation from the proposition logic to the proof language. Although the DNN model often generates syntactically and/or logically incorrect proofs, we find that they are useful as a guide of proof synthesis. The second part is to introduce a DNN model, which we call a proposition-to-proof model, specialized to proof synthesis. To this end, we make a statistical model for proof synthesis, show that the statistical model can be decomposed to fine-grained probability distributions, and design the proposition-to-proof model to approximate the fine-grained distributions.
We also empirically confirmed that the proposition-to-proof model outperforms the off-the-shelf model in the first part for proof synthesis.
Contact:
David SPRUNGER < sprunger [at] nii.ac.jp >
SPECIAL
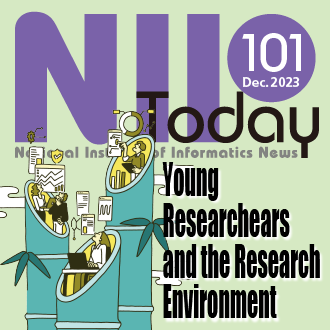
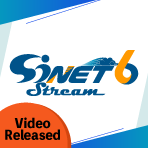
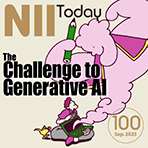
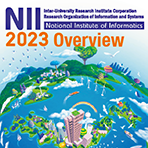
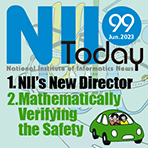
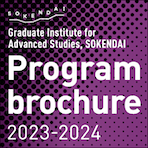
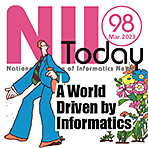
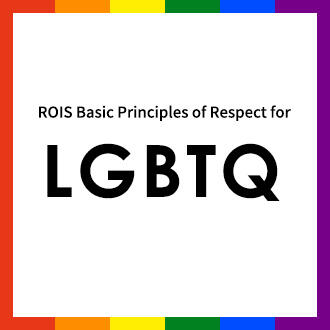
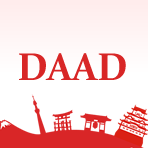